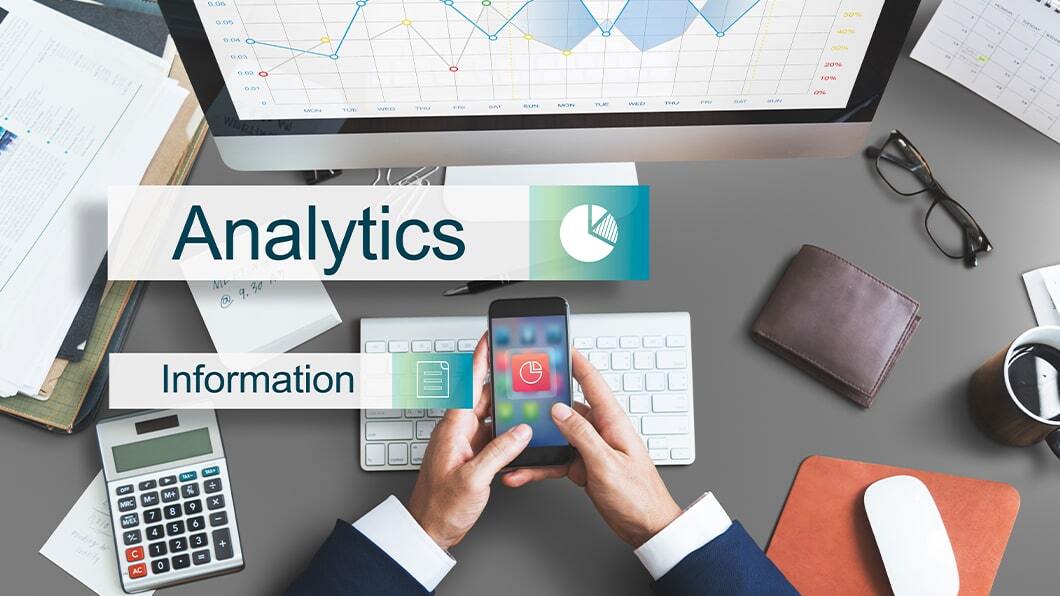
Edge Analytics: Real-Time Data Processing at the Edge
Introduction
In today’s rapidly evolving technological landscape, the exponential growth of data has presented both challenges and opportunities. The sheer volume, velocity, and variety of data generated by numerous devices and sensors have paved the way for new approaches to data processing and analysis. One such approach that has gained significant traction is edge analytics. Edge analytics involves processing and analyzing data at or near the edge of the network, closer to the data source, enabling real-time insights and immediate actions. In this blog, we will explore the concept of edge analytics and its potential benefits across various industries.
Understanding Edge Analytics
Edge analytics refers to the practice of performing data processing, analysis, and inference at the edge of a network, often on edge devices or gateways, rather than relying solely on centralized cloud or data center infrastructures. By processing data locally, closer to where it is generated, edge analytics reduces the latency and bandwidth requirements associated with transmitting data to the cloud for processing. This approach enables near-real-time analysis and decision-making, making it particularly valuable in scenarios where timely responses are critical.
Advantages of Edge Analytics
- Reduced Latency: By processing data at the edge, near the data source, edge analytics significantly reduces the time required for data to travel to the cloud and back. This reduced latency enables organizations to derive insights and make decisions in real-time, leading to faster response times and improved operational efficiency.
- Bandwidth Optimization: Transmitting vast amounts of data from edge devices to the cloud for processing can strain network bandwidth. With edge analytics, data is processed locally, and only relevant insights or aggregated results are sent to the cloud. This optimization of bandwidth utilization not only reduces costs but also ensures more efficient utilization of network resources.
- Enhanced Data Privacy and Security: Edge analytics addresses concerns related to data privacy and security by minimizing the transmission of sensitive data over the network. Since data processing takes place locally, organizations can retain greater control over their data and minimize the risks associated with transmitting sensitive information to the cloud.
- Offline Operation: In scenarios where a reliable network connection may not be available or practical, edge analytics enables data processing and analysis to continue offline. This capability is especially valuable in remote locations, such as offshore oil rigs or rural areas, where maintaining connectivity to a central cloud infrastructure can be challenging.
Applications of Edge Analytics:
- Industrial IoT: Edge analytics is revolutionizing the Industrial Internet of Things (IIoT) by enabling real-time monitoring and analysis of sensor data in manufacturing plants, oil refineries, power grids, and other industrial settings. By processing data at the edge, machine failures can be predicted, maintenance schedules optimized, and anomalies detected promptly, leading to improved operational efficiency and reduced downtime.
- Smart Cities: Edge analytics plays a crucial role in building smart cities. By processing data from various sources such as traffic cameras, environmental sensors, and energy grids, edge analytics can provide real-time insights for efficient traffic management, environmental monitoring, and resource optimization.
- Healthcare: In healthcare, edge analytics enables the processing and analysis of patient data at the point of care. Medical devices, wearables, and sensors can collect and analyze data in real-time, facilitating early disease detection, personalized treatment plans, and improved patient outcomes.
- Retail: Edge analytics is transforming the retail industry by enabling personalized customer experiences and optimizing inventory management. By analyzing data from in-store sensors, beacons, and video feeds, retailers can deliver targeted promotions, optimize store layouts, and ensure efficient supply chain management.
Edge analytics represents a paradigm shift in data processing and analysis, empowering organizations to harness the power of real-time insights at the edge of their networks. By leveraging edge computing capabilities, businesses can overcome the limitations of traditional cloud-centric approaches, reduce latency and bandwidth constraints, enhance data privacy and security, and enable offline operation in remote or unreliable network environments. The applications of edge analytics span across various industries, including industrial IoT, smart cities, healthcare, and retail, bringing significant benefits and transformative opportunities.
As edge analytics continues to evolve, advancements in edge computing technologies, such as increased processing power and machine learning capabilities at the edge, will further enhance its potential. With the proliferation of edge devices and the advent of 5G networks, the volume of data generated at the edge is expected to soar. Edge analytics will play a pivotal role in efficiently managing and extracting value from this data deluge.
However, it is important to acknowledge that edge analytics is not a replacement for cloud-based analytics but rather a complementary approach. While edge analytics offers real-time processing and immediate actions at the edge, cloud-based analytics provide the scalability, centralized storage, and advanced analytics capabilities necessary for in-depth analysis and long-term insights.
Organizations considering implementing edge analytics must carefully evaluate their specific use cases, infrastructure requirements, and business objectives. Factors such as the complexity of data processing algorithms, the need for real-time decision-making, and the availability of reliable network connectivity should be taken into account. Additionally, ensuring data security, privacy, and compliance with relevant regulations is crucial when adopting edge analytics solutions.
In order to effectively implement edge analytics, organizations need to consider a few key factors. Firstly, selecting the right edge analytics platform or solution is crucial. There are numerous providers in the market offering a range of edge computing and analytics capabilities. Organizations should evaluate the scalability, compatibility, and ease of integration with existing systems when choosing a solution.
Secondly, data management and governance are essential aspects of edge analytics. Organizations must establish robust data collection, storage, and processing mechanisms at the edge. This involves defining data ingestion protocols, implementing data filtering and preprocessing techniques, and ensuring data quality and integrity. Additionally, clear data governance policies should be put in place to address issues such as data ownership, access control, and regulatory compliance.
Furthermore, edge analytics necessitates a shift in traditional analytics workflows. Data scientists and analysts need to adapt their skills and methodologies to work effectively with edge data processing. This may involve developing or acquiring expertise in edge computing technologies, real-time analytics, and deploying machine learning models at the edge. Collaborative efforts between data scientists, domain experts, and IT professionals are crucial for successful implementation.
It’s worth noting that as edge analytics gains prominence, challenges may arise. The distributed nature of edge computing introduces complexities in managing and maintaining edge devices and gateways. Ensuring consistent performance, reliability, and security across a diverse set of edge nodes can be demanding. Organizations must establish effective monitoring and management systems to address these challenges and ensure the smooth operation of edge analytics infrastructure.
In conclusion, edge analytics represents a significant advancement in real-time data processing and analysis. By leveraging edge computing capabilities, organizations can unlock immediate insights, reduce latency, optimize bandwidth usage, and enhance data privacy and security. The applications of edge analytics span across various industries, empowering businesses to make faster decisions, optimize operations, and deliver superior customer experiences. However, successful implementation requires careful consideration of infrastructure, data management, skill sets, and ongoing maintenance. With the right approach, organizations can harness the power of edge analytics to gain a competitive edge in the data-driven era.
FAQ’s?
1. What is the difference between edge analytics and cloud analytics?
Edge analytics involves processing and analyzing data at or near the edge of the network, closer to the data source. It enables real-time insights and immediate actions. On the other hand, cloud analytics refers to processing and analyzing data in a centralized cloud infrastructure. While edge analytics offers low latency and offline capabilities, cloud analytics provides scalability, advanced analytics, and centralized storage.
2. What are the benefits of edge analytics?
Edge analytics offers several advantages, including reduced latency, optimized bandwidth usage, enhanced data privacy and security, and offline operation. It enables organizations to make real-time decisions, improves operational efficiency, and allows for faster response times.
3. In which industries can edge analytics be applied?
Edge analytics has applications in various industries. It is commonly used in industrial IoT for real-time monitoring and predictive maintenance. It is also utilized in smart cities for traffic management and resource optimization. Other sectors that benefit from edge analytics include healthcare for patient monitoring, and retail for personalized customer experiences and inventory management.
4. What are the key considerations for implementing edge analytics?
When implementing edge analytics, organizations should consider factors such as selecting the right edge analytics platform or solution, establishing robust data management and governance practices, adapting analytics workflows, and addressing challenges related to managing and maintaining edge devices and gateways.
5. Can edge analytics work offline?
Yes, edge analytics can operate offline. Since data processing and analysis occur at the edge, organizations can continue to derive insights and take actions even in scenarios where a reliable network connection is not available or practical.